Munjal Shah’s Hippocratic AI developed Polaris, the first safety-focused large language model constellation for real-time patient-generative artificial intelligence healthcare conversations.
Unlike prior LLM works in healthcare, which focus on tasks like question answering, Hippocratic AI’s work specifically focuses on long multiturn voice conversations. Healthcare has been considered both an ideal use case and a daunting challenge for artificial intelligence researchers. AI in medicine has the potential to alleviate crushing staffing shortages, democratize access to care, and improve patient outcomes.
However, achieving these ends requires rigorous training of the system so that it expresses essential capabilities such as rapport building, trust building, empathy and bedside manner augmented with advanced medical reasoning.
Founded in early 2023, Hippocratic AI is optimizing the benefits of generative AI in healthcare while avoiding the risk that comes with diagnostic applications. Instead of using AI to diagnose, says Munjal Shah, the company’s co-founder and CEO, Hippocratic AI is designing generative AI agents to carry out nondiagnostic assistance through empathetic patient conversations that support healthcare delivery at scale.
Shah describes the vision behind this approach: “We said, ‘What if, instead of co-pilot, we do autopilot? What if we build fully automated Gen AI agents that call people on the phone and talk to them, that do preoperative calls before your colonoscopy to make sure you’re taking the drink and check in with you to make sure you’re getting that MRI you were supposed to get done that you keep blowing off?’”
At the core of the company’s approach is Polaris, a novel, safety-focused LLM constellation architecture for healthcare.
“I saw ChatGPT and I said, ‘Oh, the AI I’ve been waiting for my whole life has arrived,’” says Shah, who has a master’s degree in AI from Stanford and spent his early career building startups that eventually sold to Google and Alibaba.
“Then I said, ‘I’ve just spent a decade wandering in healthcare and learned a ton about healthcare,’” he continues. “And I realized, actually, my second mission in life probably just found me, which is that I have the right set of experiences to pull these two things together.”
But how exactly does one train an AI to have truly beneficial healthcare conversations? Hippocratic AI’s process offers a window into the cutting edge of AI safety.
The Polaris Constellation: A Safety-Focused Constellation Architecture for Healthcare
Unlike general-purpose AI assistants, Polaris was built from the ground up to excel at extended, multiturn voice conversations in healthcare settings. It’s what Hippocratic AI calls a “constellation architecture” — not one monolithic model, but a trillion-parameter constellation system capable of carefully choreographed interactions between a primary and multiple specialist LLMs.
At the heart of the system is a primary agent that drives the overall flow of the conversation, aiming to build rapport and trust with patients.
But the primary agent doesn’t work alone. It’s supported by a fleet of smaller models that Hippocratic AI terms “specialist support agents.” These narrowly focused AIs handle specific patient interactions — things like preop or postop check-ins, medication management, lab result analysis, or nutrition advice. In many cases, these interactions don’t happen at all; in others, a generative AI agent can free up human healthcare staff for higher-value patient interactions by alleviating the burden of often time-consuming nondiagnostic work that can be difficult to manage when understaffed.
This distributed approach offers several advantages. The specialist models can be more easily updated or swapped out as medical knowledge evolves or new use cases emerge. It also introduces multiple layers of safety checks, with built-in guardrails to catch potential errors from the primary model.
Training for Empathy
Training an AI to convincingly emulate healthcare professionals requires far more than simply ingesting medical textbooks. Hippocratic AI’s process involves multiple stages, each designed to instill different capabilities into the model.
The foundation is built on a massive corpus of high-quality medical data. This includes published research and drug databases, but also more applied sources like clinical care plans, regulatory documents, and detailed medical reasoning examples. The goal at this stage is to give the model a comprehensive grounding in medical knowledge and terminology.
But knowledge alone doesn’t make an empathetic conversationalist. The next crucial ingredient is dialogue — lots and lots of dialogue. Hippocratic AI generated a vast dataset of simulated conversations with the help of registered nurses and patient actors. These weren’t simple Q&A sessions; they were rich, multiturn interactions designed to mirror the complexities of real-world patient encounters.
The company also created a diverse set of fictional patient profiles to train the LLMs. Each of these virtual patients came complete with detailed medical histories, current medications, recent lab results, and even personality quirks.
This dataset allowed the AI to practice navigating human elements that often complicate medical discussions, like a patient reluctant to discuss lifestyle changes or one who easily veers off on tangents.
The Power of Context
One of the key challenges in training an AI for extended conversations is maintaining coherence and context over many turns of dialogue.
Rather than treating each conversation as a single training example, Hippocratic AI broke them down into individual turns. The model’s system prompt — essentially its working memory and instructions — was continuously updated based on the conversation history, current objectives, and any relevant information from the specialist support agents.
This mimics the way a human healthcare provider might mentally update their approach as a conversation progresses. It allows the AI to be more dynamic, adjusting its communication style or level of detail based on how the patient responds.
Refining the Model With Real-World Feedback
While simulated conversations provide a strong starting point, there’s no substitute for real-world testing when it comes to high-stakes applications like healthcare. Hippocratic AI’s Gen AI agents have undergone detailed evaluations involving over 1,000 licensed nurses and 130 physicians.
These healthcare professionals engaged with the Gen AI agents in a variety of scenarios, rating its performance on crucial metrics like medical safety, patient education effectiveness, conversational quality, and “bedside manner.” The process serves as a rigorous test and generates valuable data for continuous improvement.
Munjal Shah emphasizes the importance of this reinforcement learning from human feedback, or RLHF, approach.
“We’ve actually put 1,000 nurses interacting with our large language model as if they’re patients and it’s the chronic care nurse,” he says. “And these are real licensed, registered nurses who will basically do a blind taste test where they don’t know if they’re talking to a human or a Gen AI agent. And only when they think it’s safe will we launch it.”
Safety Through Specialist Agents
While the primary conversational agent is impressive in its own right, much of Polaris’ power comes from its constellation of specialist support agents. These narrowly focused models act as a system of checks and balances, each bringing deep expertise to a specific domain of healthcare interactions.
Take, for example, the privacy and compliance specialist. In an era of stringent healthcare data regulations, this agent ensures that patient identity is verified before any sensitive information is shared. It acts as a constant guardian against potential privacy breaches.
The medication specialist is another crucial safeguard. This agent cross-checks medication names, dosages, and potential interactions. If the primary agent suggests something that could be unsafe, the medication specialist can intervene, either providing a correction or flagging the interaction for human review.
Other specialist agents handle tasks like guiding conversations through complex checklists, analyzing lab results in the context of a patient’s history, or providing tailored nutritional advice. There’s even a human intervention specialist, designed to recognize situations where the AI system may be out of its depth and seamlessly loop in a human healthcare professional to take over the conversation.
Scaling Empathetic AI
While Hippocratic AI’s approach is promising, we are only in the beginning stages of an evolutionary process. One of the most fundamental challenges is scale: How will the nuanced, empathetic communication style achieved in controlled tests hold up when deployed to millions of patients?
Hippocratic AI is actively engaging with these issues. Its use of diverse patient profiles in training data and ongoing real-world testing with a broad range of healthcare professionals is part of an awareness of the need for inclusive development. The company recently entered Phase 3 of its testing, which involves over 40 health system and payer partners testing its product internally, along with an increase to more than 5,000 nurses and 500 doctors engaged in RLHF training.
The company has also emphasized the role of its AI as an augmentation to, rather than a replacement for, human healthcare providers.
“We realize that in a sense, there’s a vision here that says, ‘What if 350,000,000 Americans all had healthcare workers to help them? Would we get better outcomes?’ Of course we would,” says Shah. “Now, generative AI working with human healthcare professionals makes it possible.”
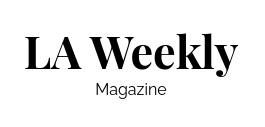
We share information about current trends and stories of people all around the world.